How Machine Learning is Optimizing Inverter Performance for Maximum Efficiency
How Machine Learning is Optimizing Inverter Performance for Maximum Efficiency. In the world of renewable energy, particularly in solar power systems, inverters play a crucial role in converting direct current (DC) electricity from solar panels into alternating current (AC) electricity that can be used in homes and businesses. However, to ensure that the energy conversion is as efficient as possible, the performance of inverters needs to be continuously monitored and optimized. This is where machine learning (ML) comes into play, transforming how inverters are managed, operated, and maintained.
The Power of Machine Learning in Energy Systems
Machine learning, a subset of artificial intelligence, involves algorithms that allow systems to learn from data and improve over time without explicit programming. By leveraging vast amounts of real-time and historical data, machine learning helps optimize the performance of inverters, pushing them towards their peak efficiency levels. This can be achieved through predictive analytics, fault detection, and performance optimization, all of which are powered by machine learning models.
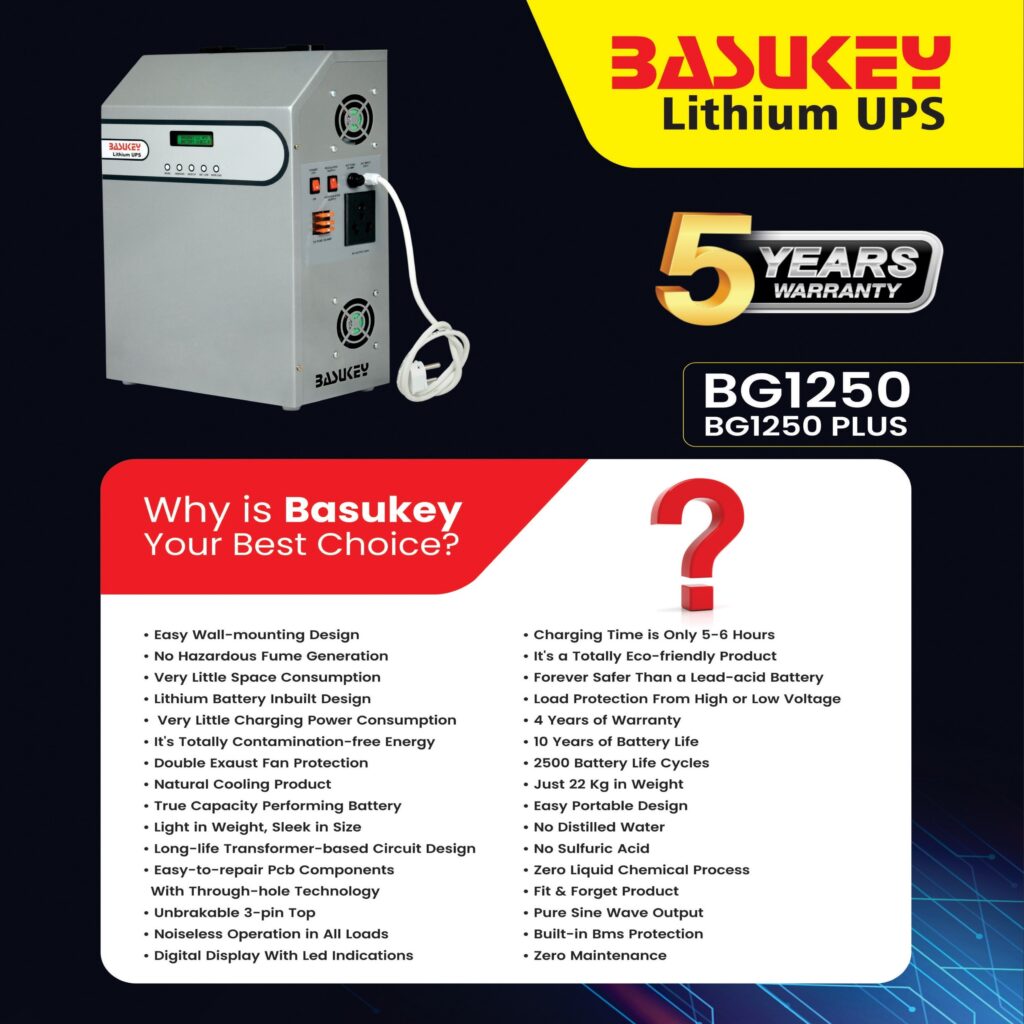
Real-Time Monitoring and Adaptive Control
One of the most significant applications of machine learning in inverter systems is real-time monitoring and adaptive control. Traditionally, inverters operate based on fixed settings and predetermined algorithms. While this works in most cases, it does not account for the variations in environmental conditions. Such as temperature, humidity, or light levels, that can affect an inverter’s performance.
Machine learning models can continuously analyze data from sensors installed on the inverter and surrounding environment. Allowing them to adjust operational parameters in real-time. For example, if the temperature increases or the sunlight intensity changes. The inverter can automatically optimize its settings for maximum efficiency. This dynamic control allows the system to adapt to fluctuations and always perform at its best. Ensuring that energy conversion is as efficient as possible.
Predictive Maintenance for Inverter Health
Inverters, like all electronic devices, can experience wear and tear over time. Faults, such as power losses or component degradation, can significantly impact their efficiency. Machine learning models are capable of detecting these issues early, allowing for predictive maintenance and minimizing downtime.
By continuously analyzing data such as voltage, current, and thermal information. Machine learning algorithms can identify patterns that signal impending failure or suboptimal performance. For example, the system can predict when certain components might need servicing or replacement, reducing the chances of a sudden breakdown. This approach extends the inverter’s lifespan, improves its reliability, and ensures a more consistent energy output.
Fault Detection and Diagnosis
Inverters can be prone to faults such as grid connection issues, component malfunction. Or even inefficiencies due to external factors like shading or soiling of solar panels. Detecting these issues early can prevent a significant loss in energy production. With machine learning, advanced fault detection algorithms can be employed to continuously monitor the inverter’s output and quickly identify any discrepancies from normal operation. -For instance, if the inverter starts underperforming, ML systems can instantly flag the issue. Diagnose whether it’s a hardware malfunction, or if environmental factors such as dirt on the panels are affecting performance. Such insights help reduce downtime and improve operational efficiency by quickly addressing the root causes of issues.
Energy Yield Optimization
Maximizing the energy yield is one of the primary goals of a solar power system. Machine learning algorithms can assist in forecasting energy production and optimizing inverter performance by adjusting settings based on weather forecasts, load demand, and solar panel condition.
Using predictive models, inverters can adjust their operation to account for factors like cloud cover or predicted weather patterns. By proactively adapting to these environmental conditions. Inverters can optimize the energy they convert from the solar panels, leading to a better return on investment for solar energy systems.
Enhancing Grid Integration
Modern solar energy systems are often connected to the grid, which can have its own fluctuating demands. Machine learning can improve the integration of inverters with the grid by predicting power consumption patterns and adjusting inverter outputs accordingly.
For example, during peak demand times when the grid requires more energy. The inverter can automatically adjust its output to ensure a stable energy flow to the grid. Conversely, during low demand, it can regulate its output to prevent overloading. This dynamic interaction improves grid stability and makes solar energy a more reliable resource.
Conclusion
Machine learning is undeniably transforming the way we optimize inverter performance. Through real-time adjustments, predictive maintenance, fault detection, and energy yield optimization, ML ensures that inverters work at maximum efficiency. This not only improves the overall performance of solar power systems but also contributes to the broader goal of sustainable energy generation. As machine learning continues to evolve, we can expect even more sophisticated and efficient inverter technologies, revolutionizing the renewable energy landscape for years to come.